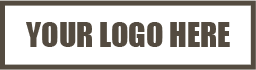
How machine learning helps drug development
- Rating:
-
- (0 / 5)
Staffcontrolling.com: An Intelligent System for Remote Employee Monitoring
Information Technology: A Powerful Engine of Modern Progress
Information Technology: A Driving Force of Modern Progress
Viritia IT Company: Driving Innovation with Cutting-Edge Technology
Videoliedetector.com - Empowering Truth through Advanced Technology
Robobroker.com - Empowering Your Stock Trading Experience
Masterdeal.com - Your Ultimate Online Marketplace
Servicingmarket.com - Connect with Top Performers Worldwide
Knowledgehunting.com - Unlock Your Potential with Gray Hair Experts
Repairingmarket.com - Your Smart Solution for Repair Services Worldwide
Oemmanufacture.com - An Online Platform for Contract Manufacturers Worldwide
Corphiring.com - Redefining Recruitment with Video Insights
Rentingmarket.com - Revolutionizing Global Property Rentals
Viritia IT Solutions - Rndoutsourcing.com
Viritia IT Solutions - Massmediamarket.com
Viritia IT Solutions - Staffcontrolling.com
Viritia IT Solutions - Our Innovative Projects
Empowering Efficiency and Innovation through AI Technology | Viritia IT Company
Quantum Internet - Fantastic Opportunities Get Closer
"Face Index". In the United States create a system to spy on every inhabitant of the Earth
Where are you, my digital twin? Started with the brain
Artificial intelligence in the modern world
Types of artificial intelligence
Will there be a "quantum apocalypse"?
3 Instagram Content Ideas
Quantum teleportation and fiber optic Internet
Top 10 IT professions of the future and present
How a new programming language will speed up computers
Most Popular Programming Languages
Microsoft experiments with Rust
Microsoft and memory management. Will a new programming language solve security problems?
Initial Public Offering (IPO)
Venture funds providing seed capital
Our top tips for entrepreneurs
Credit or venture capital
How to get venture capital
Venture funding
Venture Capital
Sources of venture capital
Minimization of financial risks in the process of venture financing of innovative projects
Minimization of financial risks in the process of selecting entrepreneurial projects for venture financing
Characteristic features of venture financing
Prerequisites for the emergence of venture financing mechanisms
Venture Capital in Central and Eastern Europe
Estimation of the investor's share in the company in venture investment
When to borrow and when to share?
Methods of financing investment projects
How to find your niche on the internet
Direct investment as a source of financing
Expertise of innovative projects